In medical imaging, radiomic features make it possible to characterize heterogeneity of a region of interest at the anatomical level. This way of quantifying the heterogeneity of a region of interest can be useful, for example, in order to identify the more aggressive tumors in oncology. To do this, we hypothesize here that variation in magnetic resonance imaging (MRI) acquisition sequences and its resulting different levels of contrast would make it possible to optimize the subsequent radiomic analysis.
Open Science available to everyone!
Know moreMcMedHacks: Week 1/Introduction to classical machine learning
Know moreNursing Data Standards: Landscape and Directions in Canada
Know moreHow to write a paper and publish it in a high ranking science journal?
Know moreSummer School 2022: Management of health services in a Franco-Quebec context
Know more89e Congrès de l'Acfas
Know more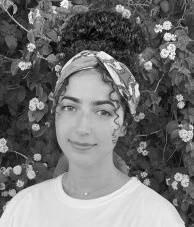
Lyna Abrougui
M.Sc. candidate
Faculté de médecine
Université Laval
Prediction and early identification of stroke is crucial to prevent emergency department (ED) revisits and initiate treatment, reducing morbidity and mortality.
This project focuses on the analysis of non-contrast brain CT (NCCT) data to predict early ED revisits for patients coming back with a stroke diagnosis. The first objective will be gathering open-source NCCT data as well as NCCT data from the Integrated Health and Social Services Center from Chaudiere-Appalaches (CISSS-CA) to classify the presence/absence of stroke using an existing model. The second objective will be to develop and test a machine learning model with weights from the previous model and other relevant clinical data to classify short-term revisits to the ED as an outcome.
From a clinical perspective, the development of such a tool may help support neuroradiologists in image interpretation and clinical decision making in the ED.
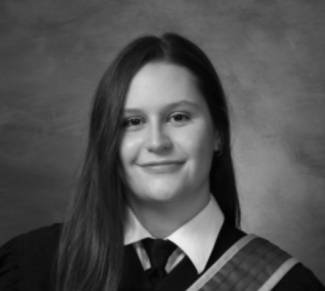
Raphaëlle Giguère
M.Sc. candidate
Faculté de médecine
Université Laval
Delirium is a condition that, when left unmanaged, is associated with increased mortality and longer hospitalization of patients in intensive care; therefore, its detection should be an integral part of care. It is characterized by confusion, anxiety and reduced alertness. It is estimated that 75% of delirium cases are not detected on admission to hospital. Detecting such an acute condition requires frequent monitoring of participants, which is labor intensive and requires expertise. However, the participants' vital signs, which can be collected continuously throughout their stay in intensive care, could contain information indicative of the present state of consciousness, and possibly predictive of the future state.
Our goal is to build an automatic machine learning classifier based on vital sign data to (a) identify times when the patient was delirious, and (b) predict delirium incipience. As a primary measure, we will use a clinically validated tool, the Confusion Assessment Method for Intensive Care Unit (CAM-ICU). This assessment was performed twice a day, once in the morning and once in the afternoon, in our study population at the CISSS de Chaudière-Appalache (Hotel Dieu de Lévis). The learning algorithm will be trained on the participants' vital signs before, after, and during the delirium episodes in order to (a) extract the vital sign characteristics related to a delirium state; (b) the probability that the patient is delirious or not, based on these characteristics; and (c) the probability that the patient will develop a delirium state within a reasonable time window (e.g. 1 hour).
Even if the machine learning model does not reach the accuracy and precision of a validated questionnaire, its use in healthcare facilities would optimize care, mainly by drawing attention to any suspicious drift (high sensitivity). Considering that patients who remain with untreated delirium are associated with higher mortality rates and longer ICU stays, a clinical indicator such as this model can help the care team manage this otherwise unnoticed symptom.
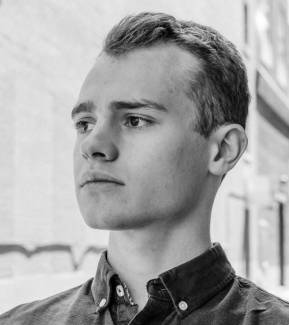
Nicolas Desjardins-Proulx
M.Sc. candidate
Medical Physics Unit
McGill University
The risk associated with the stochastic effects of neutron radiation is known to be strongly energy dependent. Over the past decade, several studies have used Monte Carlo simulations to estimate the relative biological effectiveness (RBE) of neutrons for various types of DNA damage in order to understand its energy dependence at the fundamental level. However, none of these studies implemented DNA repair simulations in their pipeline.
In this project, we investigated the effects of adding repair mechanisms to Monte Carlo-based RBE estimates of DNA damage by neutrons. Our group had previously carried out condensed history (CH) simulations to profile the energy spectrum and relative dose contribution of the secondary particles produced by neutron interactions in tissue. In this project, we use the results of our CH simulations to simulate the irradiation of TOPAS-nBio’s DNA model by a flat spectrum of neutrons ranging from 1 eV to 10 MeV, as well as reference X-rays at 250 keV. Induced DNA damage are recorded using the standard DNA damage data (SDD) format abd DNA repair are simulated using the DNA Mechanistic Repair Simulator (DaMaRiS) framework.
Discover
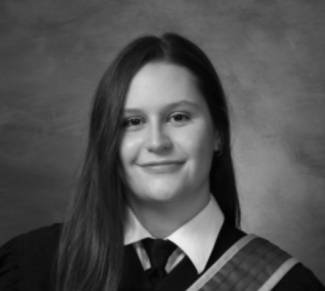
Featured project
Delirium is a condition that, when left unmanaged, is associated with increased mortality and longer hospitalization of patients in intensive care; therefore, its detection should be an integral part of care. It is characterized by confusion, anxiety and reduced alertness. It is estimated that 75% of delirium cases are not detected on admission to hospital. Detecting such an acute condition requires frequent monitoring of participants, which is labor intensive and requires expertise.