Aucun contenu de page d'accueil n'a été créé pour l'instant.
Discover
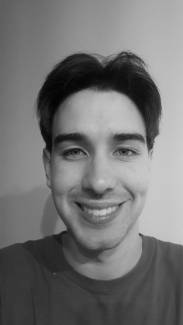
Featured project
Prostate cancer is the second most frequent cancer and the fifth leading cause of cancer death among men. To improve patient outcomes, treatment must be personalized based on accurate prognosis. Nomograms already exist to identify patients at low risk for recurrence based on preoperative clinical information, but these tools do not use patients’ medical images.