The aim of this doctoral thesis is to develop a tool able to automatically provide organs of interest segmentation in computed tomography images using machine learning techniques.
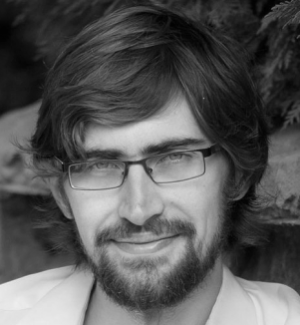
Ronan Lefol
Ph.D. candidate
Faculté des sciences et de génie
Université Laval
The aim of this doctoral thesis is to develop a tool able to automatically provide organs of interest segmentation in computed tomography images using machine learning techniques.
This tool will then be used to calculate organ doses in order to establish personalized dosimetric records in medical imaging. Doses will be calculated using informations obtained from images, radiographic technique and GPU-based Monte Carlo dose calculation algorithm (GPUMCD). Automated pipelines will be implemented to process large amounts of data.
The proposed tool provides a better evaluation of population exposure to ionizing radiation caused by medical imaging procedures.
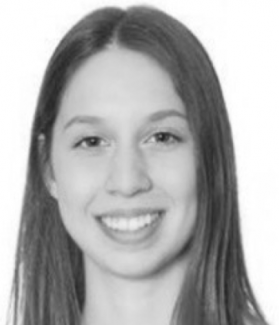
Danahé LeBlanc
M.Sc. candidate
Faculté des sciences et de génie
Université Laval
Prostate cancer is the most common form of cancer in men in Canada.
This research project aims to establish a prognosis for a patient suffering from prostate cancer as well as predict the final pathology, by predicting the presence of lymph node metastases, from a FDG PET-CT. Radiomic characteristics are defined as the process of quantitative extraction of usable high-dimensional data from medical images. These are biomarkers that are difficult to see with the naked eye, such as texture and intensity. The database is made up of 250 prostate cancer patients. After filtration, a subset of 331 radiomic characteristics was selected. The accuracy of the model is 74.5%. This corresponds to an increase in precision of 6% compared to a model trained on all the extracted characteristics.
Ultimately, the algorithm will better predict the risk of recurrent prostate cancer and help improve methods and choice of treatment.
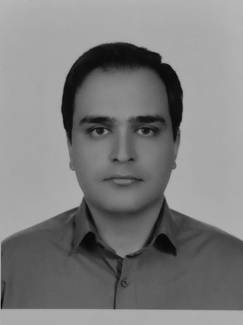
Mojtaba Safari
Ph.D. candidate
Faculté des sciences et de génie
Université Laval
The clinical and economic burden of prostate cancer in Canada is substantial and is rising. It has been indicated that 1 in 7 men will develop prostate cancer during their lifetime, and another 1 in 27 will die due to the prostate cancer. However, only a part of prostate cancer cases is clinically important which make the prostate cancer case discrimination crucial to avoid over-treatment. Compared to ultrasound imaging method, advanced MRI modalities have demonstrated a better diagnostic accuracy and is becoming a clinical routine examination for patients at risk of having clinically significant prostate cancer. Even though the version two of PI-RADS has been recently published to facilitate MRI modalities application in prostate cancer, they still present limitations. For instance, variability is reported in terms of inter-reader agreement and diagnosis accuracy, mainly depend on reader experience.
This project aims to find a machine learning based approach for predication and segmentation of intraprostatic lesions to better guide radiation treatment. For accomplishing this task, the most advanced MRI modalities including DTI-MRI and DWI-MRI along with the anatomical MRI modalities will be employed. From the quantitative MRI modalities several maps that enhance specific features of the lesion will be extracted. Then after, texture information of the MRI modalities and selected maps will be extracted. In this step machine learning methods will be employed for feature selection and classification purposes. Finally, the prostate cancer extension and its type are identified.
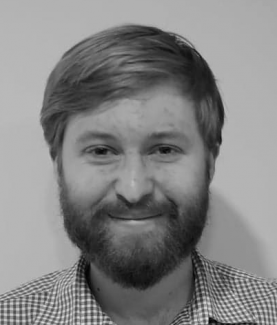
Gabriel Couture
M.Sc. candidate
Faculté des sciences et de génie
Université Laval
This project consists of establishing good practices in health data management and building a software infrastructure in order to apply them.
We have developed pipelines that allow daily recovery of brachytherapy treatment data in order to calculate and store their dosimetric indices in a database dedicated to research. These indices are essential for planning radiotherapy treatments and for estimating their quality.
The aggregation of these indices allows different researchers such as bio-statisticians and radiation oncologists to carry out studies on larger data sets.
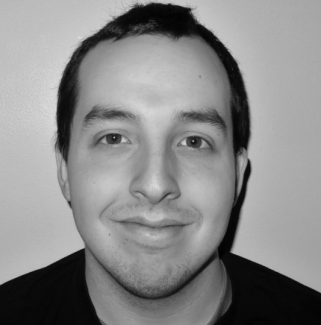
Samuel Ouellet
M.Sc. candidate
Faculté des sciences et de génie
Université Laval
The objective of this project is to extract a set of relevant data from the files produced by medical imaging devices.
The process consists of building ETL (extract-transform-load) pipelines to make the data consumable for analysis and visualization. An example of analysis consists in observing the trend in dose administered to patients according to the establishment, protocol or device used, in order to possibly identify non-standard practices.
The data extracted could also guide practice by making it possible to assess the relevance of certain studies, and thus to optimize resources in the health network.
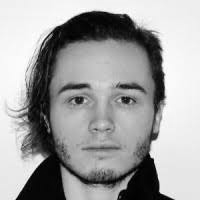
Keven Voyer
M.Sc. candidate
Faculté des sciences et de génie
Université Laval
An exception drug is a drug that is not usually covered by the public drug insurance plan (RPAM). The measures implemented at the RAMQ for exceptional drugs allow the entire population to obtain coverage for certain drugs if they are used in compliance with the indications recognized by the Institut national d'excellence en santé et services sociaux (INESSS). Exception drugs are now a large and constantly increasing part of total spending on prescription drugs.
For the RPAM, one of the ways to control this increase is to reimburse these drugs according to pre-established rules. Currently, the system automatically processes around 20% of requests while the rest are directed to a case-by-case analysis, which generates delays.
This project is to help the business sector respond more quickly to requests for approval of exception drugs. A tool will be developed based on 15 years of data collected by the current system, and will aim to increase the number of requests processed automatically.
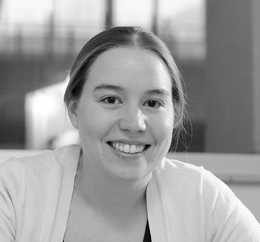
Anne-Sophie Charest
Associate Professor
Faculté des sciences et de génie
Université Laval
Anne-Sophie Charest is an associate professor in the Department of Mathematics and Statistic at Université Laval. She is the director of the certificate and bachelor programs in statistic.
She holds a bachelor degree in probability and statistic from McGill University (2007) and a doctor degree in statistic from Carnegie Mellon University, in Pittsburgh (Pennsylvania, 2012).
Her research interests are focused on statistical data confidentiality including synthetic data set creation and differential confidentiality criterion, survey data analysis, treatment of missing data and the Bayesian inference approach.
Anne-Sophie Charest is a regular member at the Big Data Research Center (BDRC)-Université Laval and the Mathematics Research Center (MRC)-Université de Montréal.
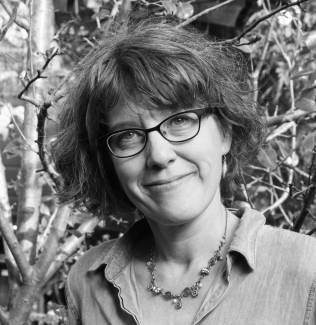
Isabel Fortier
Assistant Professor
Faculté de médecine
McGill University
Dr. Isabel Fortier is researcher at the Research Institute of the McGill University Health Centre (RI-MUHC) where she leads the Maelstrom Research program.
This program aims to provide the international research community from diverse disciplines with resources (expertise, methods, and software) to leverage and support data harmonization and integration across studies. The Maelstrom Research team develops methods and software, conducts methodological research, generates comprehensive catalogues of study metadata, and creates infrastructures supporting data management, harmonization and co-analysis.
The team is collaborating on or leading the data cataloguing and harmonization activities of a number of national and international projects including IALSA (Integrative Analysis for Longitudinal Study Analysis, more than a 100 studies), ReACH (Research Advancement through Cohort Cataloguing and Harmonization, 26 studies) and CPTP (Canadian Partnership for Tomorrow Project, 5 studies).
Ultimately, Maelstrom Research resources help to optimize the use of existing research data, enhance capacity for national and international collaborative and cross-disciplinary research (outputs generated faster and at lower cost), and improve quality of research practices.
Discover
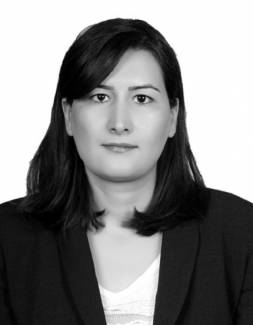
Featured project
This research project is based on the analysis of massive data on the NOL index and other intraoperative clinical parameters used by anesthesiologists during surgery. These parameters help them make analgesic treatment decisions in a non-communicating patient under general anesthesia and in whom it is impossible to assess pain and analgesic needs by standard questionnaires performed on awake patients.
First, the objective is to interpret the values of this index in relation to the decisions made by the clinician.