Radiotherapy treatments currently used in the clinical field are rarely modified. They generally consist of a global therapy of 50 grays, fractionated in five treatments of two grays every week for five weeks.
Thus, it could be worthwhile to develop a numeric tool, based on mathematical models found in the literature, in order to compare different types of treatment without having to test them on real tissues. Several parameters are known to alter the tissue response after irradiation including oxygen
The first objective of the project is to design efficient convolutional network classification models (CNNs) using mass spectrometry data (1D and 2D) for clinical diagnosis (cancer and infection).
Once finalized, the second objective is the interpretation of these classification models in order to identify spectral regions of interest that may correspond to new diagnosis or therapeutic biomarkers.
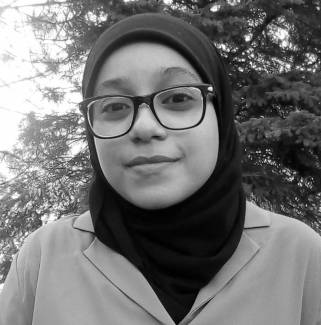
Oumaima Ouffy
M.Sc. candidate
Faculté des sciences et de génie
Université Laval
Il est souvent difficile de partager des données dénominalisées entre différentes organisations et chercheurs en raison de contraintes éthiques liées à la confidentialité des répondants. Il peut ainsi s’écouler de longs mois, parfois même des années, entre la rédaction d’un projet de recherche et le début de l’analyse planifiée, ce qui limite la capacité des chercheurs à mener des travaux scientifiques de pointe au moment opportun et contribue à allonger inutilement la formation d’étudiants gradués, entre autres problèmes. Une solution possible est de créer un jeu de données synthétiques à partager aux chercheurs en attente de l’accès au jeu de données original. Ce jeu de données synthétique serait représentatif des données originales, mais créé de façon à ne pas révéler d’information confidentielle sur les répondants. Il permettrait aux chercheurs de se familiariser à l’avance avec les variables mesurées, d’anticiper les difficultés techniques du projet de recherche (stockage, logiciels, gestion des accès), et de planifier de meilleurs protocoles de recherche.
Nous étudions ici les enjeux techniques liés à la création de tels jeux de données synthétiques dans le domaine de la santé. Il faut notamment s’assurer que les modèles statistiques utilisés soient assez flexibles pour bien modéliser les corrélations entre les variables collectées, tout en s’assurant de ne pas sur-ajuster ceux-ci, ce qui pourrait nuire à la protection de la confidentialité. Le travail s’articulera autour de la création d’un jeu synthétique pour un sous-ensemble des données collectées par le Consortium d’identification précoce de la maladie d’Alzheimer - Québec (CIMA-Q), pour qui le partage des données à la communauté de recherche sur la maladie d’Alzheimer canadienne et internationale est un objectif important.
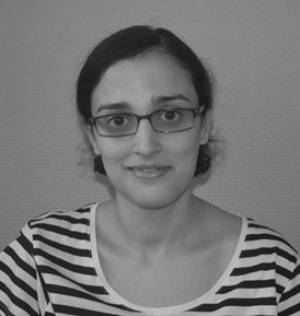
Khawla Seddiki
Ph.D. candidate
Faculté de médecine
Université Laval
The first objective of the project is to design efficient convolutional network classification models (CNNs) using mass spectrometry data (1D and 2D) for clinical diagnosis (cancer and infection).
Once finalized, the second objective is the interpretation of these classification models in order to identify spectral regions of interest that may correspond to new diagnosis or therapeutic biomarkers.
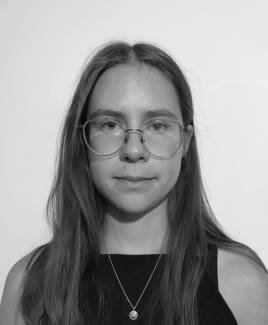
Corinne Chouinard
Undergraduate intern
Faculté des sciences et de génie
Université Laval
Radiotherapy treatments currently used in the clinical field are rarely modified. They generally consist of a global therapy of 50 grays, fractionated in five treatments of two grays every week for five weeks.
Thus, it could be worthwhile to develop a numeric tool, based on mathematical models found in the literature, in order to compare different types of treatment without having to test them on real tissues. Several parameters are known to alter the tissue response after irradiation including oxygen
partial pressure in irradiated regions, particle type hitting the tissue, and treatment duration.
The Python code created as the main part of the project is intended to facilitate the optimization of radiotherapy treatment by generating graphs showing cell survival after a certain number of fractions, taking many parameters into account. When completed and integrated to a graphical interface, the code will be easy to use and helpful for ongoing research projects.
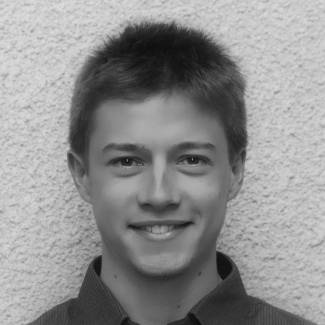
Thibaud Godon
Ph.D. candidate
Faculté des sciences et de génie
Université Laval
Metabolomics is one way of studying metabolism. The presence of certain metabolites, or the breakdown of metabolic pathways can serve as indicators of a patient's health. They can serve as markers for certain diseases such as cancers, or provide information on the quality of an individual's diet. Untargeted metabolomics acquisition methods produce large data matrices. The aim is to develop machine learning methods specifically suited to handle high dimensional data sets. For example models based on decision rules.
The purpose of these models being the search for biomarkers, they must be sparse in order to be able to be interpreted by a human expert. We also try to develop new approaches to better interpret some existing and efficient models. Interpretability is essential in the application of machine learning to health. Models cannot be diagnostic black boxes but rather analytical tools available to experts to better understand human metabolism.
Metabolomics is one way of studying metabolism. The presence of certain metabolites, or the breakdown of metabolic pathways can serve as indicators of a patient's health. They can serve as markers for certain diseases such as cancers, or provide information on the quality of an individual's diet. Untargeted metabolomics acquisition methods produce large data matrices. The aim is to develop machine learning methods specifically suited to handle high dimensional data sets. For example models based on decision rules.
It is often difficult to share denominalized data between different organizations and researchers due to ethical constraints related to respondent confidentiality. This is a common reality in the healthcare field, given the inherent sensitivity of this type of data. One option in this case is not to share the data directly, but rather to provide access to it via a tool that controls the risk of disclosure of the queries made and allows only those it considers safe.
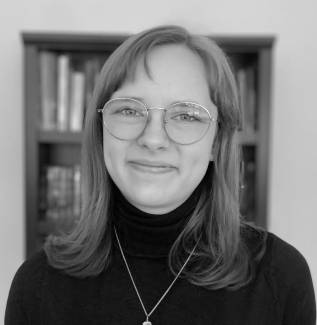
Ariane Boivin
M.Sc. candidate
Faculté des sciences et de génie
Université Laval
It is often difficult to share denominalized data between different organizations and researchers due to ethical constraints related to respondent confidentiality. This is a common reality in the healthcare field, given the inherent sensitivity of this type of data. One option in this case is not to share the data directly, but rather to provide access to it via a tool that controls the risk of disclosure of the queries made and allows only those it considers safe. DataSHIELD is such a tool which has been proposed to protect the confidentiality of a dataset, and which can be used via the statistical software R. It also allows statistical analysis on several datasets hosted in different locations, always ensuring the confidentiality of the respondents.
In this project, we are interested in the confidentiality guarantees provided by the software, and in its limitations. In particular, we wish to establish principles to guide the choice of disclosure control parameters offered with the tool, and to understand more precisely the impact of these controls on the quality of the descriptive statistics, linear models and graphs produced.
Discover
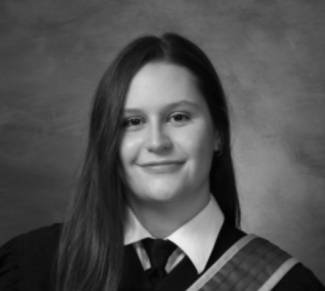
Featured project
Delirium is a condition that, when left unmanaged, is associated with increased mortality and longer hospitalization of patients in intensive care; therefore, its detection should be an integral part of care. It is characterized by confusion, anxiety and reduced alertness. It is estimated that 75% of delirium cases are not detected on admission to hospital. Detecting such an acute condition requires frequent monitoring of participants, which is labor intensive and requires expertise.