I work on improving the services of the PARADIM platform, which focuses on the annotation, reuse and analysis of medical images. The objective is to develop and optimize existing functionalities to have effective management and analysis of medical images. This involves the integration of new tools and extensions, to improve the platform's functionality and the user experience.
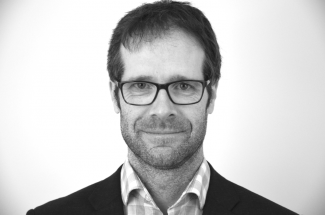
Philippe Després
Professor
Faculté des sciences et de génie
Université Laval
Philippe Després is a full professor in the Department of Physics, Engineering Physics and Optics and member of the Cancer Research Center of Université Laval, medical physicist at CHU de Québec and a regular researcher at its affiliated Research Center, membrer of the Institute intelligence and data of Université Laval and member of the Researcher Council of the New Digital Research Infrastructure Organization (NDRIO). He is the designated principal investigator of the RHHDS program.
He was trained at Université Laval (MSc 2000, Physics), Université de Montréal (PhD 2005, Physics) and University of California, San Francisco (postdoc 2005-2007, Biomedical Engineering, Molecular Imaging).
Professor Philippe Després is involved in several projects encompassing hardware and software aspects of medical imaging modalities, notably low-dose X-ray imaging, advanced imaging techniques, and solid-state detectors for molecular imaging. He pioneered high-performance computing (HPC) approaches with commodity graphics hardware (GPUs) that led to innovative applications in image processing/reconstruction and radiation dose calculations, including a fast GPU-based Monte Carlo engine to simulate energy transport in matter (GPUMCD).
As a HPC expert, professor Philippe Després is also involved in data-driven research approaches, data infrastructures and FAIR-compliant research data management. In this regard, he is responsible of biomedical data at CHU de Québec of Université Laval Research Center, the data architect of the PULSAR health research platform, the assistant director of the Big Data Research Center at Université Laval, and the co-lead of the sustainable health axis of the Observatoire international sur les impacts sociétaux de l’intelligence artificielle et du numérique (OBVIA).
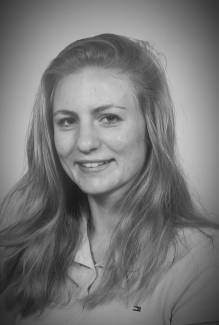
Farah Gourdel
Undergraduate intern
Faculté des sciences et de génie
Université Laval
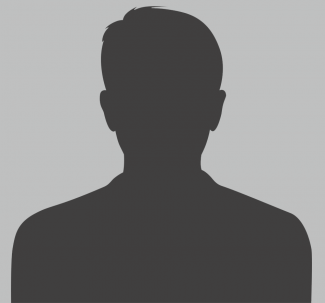
Alexandre Drouin
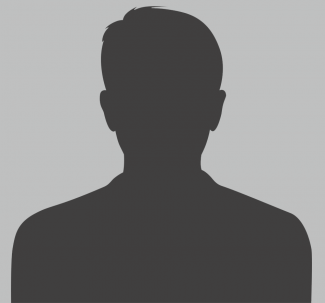
Nicolas Doyon
Faculté des sciences et de génie
Université Laval
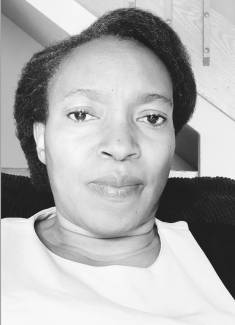
Angèle Musabyimana
Ph.D. candidate
Faculté des sciences infirmières
Université Laval
Devant la décision de faire ou non le dépistage prénatal des trisomies 21, 18 et 13, il est important que les femmes enceintes et leurs partenaires soient informés et accompagnés par leurs professionnels de la santé. Les impliquer dans la prise de décision partagée (PDP) permettrait de répondre à leurs besoins décisionnels. Aussi, l’utilisation d’un outil d’aide à la décision (OAD) faciliterait la PDP. L’objectif de ce projet est d’évaluer l’effectivité d’une intervention de mise à l’échelle d’un OAD sur le niveau d’implication des femmes enceintes et leurs partenaires dans la PDP pour le dépistage prénatal des trisomies 21, 18 et 13 (issue primaire). Ce projet sera mené dans la province de Québec et utilisera un essai randomisé par grappes et par étapes. Les principaux participants seront des services prénataux, des professionnels de la santé et des femmes enceintes et leurs partenaires. Les principales composantes de l’intervention seront une formation en ligne sur la PDP pour les professionnels de la santé et un OAD en ligne. L’issue primaire sera mesurée avec l’échelle validée SDM-Q-9 remplie par les femmes enceintes et leurs partenaires.
Les résultats de cette étude montreront l’effectivité de nos stratégies de mise à l’échelle d’un OAD pour favoriser l’adoption de la PDP.
Discover
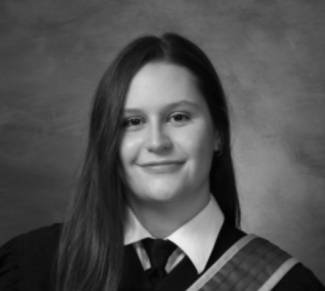
Featured project
Delirium is a condition that, when left unmanaged, is associated with increased mortality and longer hospitalization of patients in intensive care; therefore, its detection should be an integral part of care. It is characterized by confusion, anxiety and reduced alertness. It is estimated that 75% of delirium cases are not detected on admission to hospital. Detecting such an acute condition requires frequent monitoring of participants, which is labor intensive and requires expertise.