Cancer patient peer support is a beneficial tool for current patients and previous patients who can share lived experiences. However, current peer support is inefficient as it is conducted manually and relies on a coordinator to match patients based on a few known factors.
Patient portal is an emerging healthcare technology that has shown promising effects in enhancing patient care experience and promoting patient health outcomes. Opal, a digital patient-centred portal, is currently available to patients at the Cedars Cancer Center at McGill University Health Centre (MUHC), providing real-time access to personal health information (upcoming appointments, clinical notes, lab results, etc.) in conjunction with the disease- and treatment-specific education materials.
The risk associated with the stochastic effects of neutron radiation is known to be strongly energy dependent. Over the past decade, several studies have used Monte Carlo simulations to estimate the relative biological effectiveness (RBE) of neutrons for various types of DNA damage in order to understand its energy dependence at the fundamental level. However, none of these studies implemented DNA repair simulations in their pipeline.
Cancer patients go through a lot during treatment. Many patients need support from other patients who know what they are going through because family and friends, no matter how supportive they try to be, don’t always understand their struggles.
The cancer experience and the uncertainty surrounding it is anxiety provoking. One way in which the non-clinical uncertainty of the cancer experience can be reduced is through peer support. The Opal Health Informatics Group seeks to evaluate the efficacy of an artificial intelligence-based peer support matching algorithm in the pre-existing patient portal Opal in the hopes of facilitating peer support programs (for cancer patients and their caregivers) in Quebec.
La rédaction scientifique de manuscrits et d’abstracts
Know moreLa rédaction scientifique de manuscrits et d’abstracts
Know morePrediction and early identification of stroke is crucial to prevent emergency department (ED) revisits and initiate treatment, reducing morbidity and mortality.
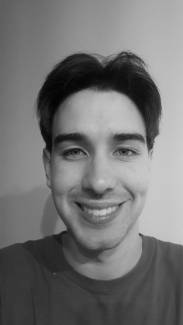
Maxence Larose
M.Sc. candidate
Faculté des sciences et de génie
Université Laval
Prostate cancer is the second most frequent cancer and the fifth leading cause of cancer death among men. To improve patient outcomes, treatment must be personalized based on accurate prognosis. Nomograms already exist to identify patients at low risk for recurrence based on preoperative clinical information, but these tools do not use patients’ medical images.
The goal of this project is to use deep learning to develop a model combining FDG-PET/CT images and patient clinical data to improve the pre-treatment prognosis of high-grade prostate cancer. This model must be efficient, but also interpretable in order to allow an expert to understand the given probabilities.
Prostate cancer is the second most frequent cancer and the fifth leading cause of cancer death among men. To improve patient outcomes, treatment must be personalized based on accurate prognosis. Nomograms already exist to identify patients at low risk for recurrence based on preoperative clinical information, but these tools do not use patients’ medical images.
Discover
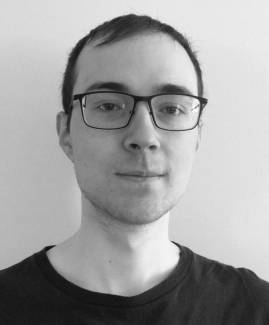
Featured project
The project consists in determining and exploring the possibilities offered by dynamic dashboards in a medical context as well as the associated data management structures. The project therefore considers several aspects of data management. In this sense, the considerations related to DICOM data transfers as well as different approaches to their management and conservation are considered. In addition, the dashboards will be designed to ensure an effective, clear and concise presentation with recognized visualization tools.