Treatment of cancer with radiation is a proven technique used worldwide. One of the ways to treat prostate cancer is by using brachytherapy either alone or as a boost. At the moment, the techniques used depend on the experience of the treatment team and researchers are trying to overcome this problem.
In our case, the technology considered to address this problem is deep learning. Therefore, the aim of this project is to use deep learning to develop tools for planning in high dose rate brachytherapy for the treatment of prostate cancer.
Four different phases are initially targeted. The first consists of a classification of treatment plans. The second is a “reinforce learning” approach to help optimize treatment plans, by modifying the optimization objectives in order to consider each patient in a unique way. The third is dose map prediction based on patient anatomy. The fourth is the generation of treatment plans; from the patient's anatomy or from a dose map to find an adequate treatment plan.
The proposed work is a new approach that will ultimately help with the planning of high dose rate brachytherapy treatments for prostate cancer.
Metabolomics is one way of studying metabolism. The presence of certain metabolites, or the breakdown of metabolic pathways can serve as indicators of a patient's health. They can serve as markers for certain diseases such as cancers, or provide information on the quality of an individual's diet. Untargeted metabolomics acquisition methods produce large data matrices. The aim is to develop machine learning methods specifically suited to handle high dimensional data sets. For example models based on decision rules.
The purpose of these models being the search for biomarkers, they must be sparse in order to be able to be interpreted by a human expert. We also try to develop new approaches to better interpret some existing and efficient models. Interpretability is essential in the application of machine learning to health. Models cannot be diagnostic black boxes but rather analytical tools available to experts to better understand human metabolism.
This research project is based on the analysis of massive data on the NOL index and other intraoperative clinical parameters used by anesthesiologists during surgery. These parameters help them make analgesic treatment decisions in a non-communicating patient under general anesthesia and in whom it is impossible to assess pain and analgesic needs by standard questionnaires performed on awake patients.
First, the objective is to interpret the values of this index in relation to the decisions made by the clinician.
The second step is to develop an artificial intelligence algorithm that can guide decision-making for greater precision and better anesthetic safety for the patient.
The characterization of Drug-Drug interactions (DDIs) is crucial for planning therapies and drugs
co-administration. While considerable efforts are spent in labor-intensive in vivo experiments and time-consuming clinical trials, understanding the pharmacological implications and adverse side-effects for some drug combinations is challenging. The joint impact of the majority of combinations remains undetected until therapies are prescribed to patients. This raises the need for computational tools predicting DDIs in order to reduce experimental costs and exhaustively characterize all drug combination effects before therapy recommendations.
Previous attempts to build such tools focused on pharmacodynamic and pharmacokinetic interactions and used features that are difficult to access in the early stages of R&D.
In this work, we propose to use data about the drugs and their targets (pathways, biomarkers, gene expressions, etc) that are available at the beginning of each drug R&D campaign. Our hypothesis is that high-level deep learning features extracted from those data will improve DDI characterization. Therefore, our models will be trained to output the pharmacological effects of DDIs as well as underlying molecular and biological pathway interactions.
Creating such a comprehensive toolkit will help to reduce risks in polypharmacy therapies.
The project focuses on the design, operationalization and validation of a sustainable health evaluation model.
This model will be adapted to a digital platform and based on solid theoretical and conceptual foundations. Furthermore, it will gather valid indicators and will be supplied by data showing a global and ecosystem conception of health.
Once operationalized, implemented and validated in a cohort study, this model will represent an innovative strategy for sustainable health through improved technologies and intervention methods.
This project studies the consequences of artificial intelligence (AI) systems and data science on public discourse, as well as their usage by the new content providers on the Web.
It will tackle the ethical aspects of learning algorithms and recommendation filters implemented by internet companies to select and present content to the user. Specifically, the project investigates the consequences of such algorithms on public health, especially in the propagation of medical misinformation and pseudo-medicine.
This project aims at taking a critical oversight on data science techniques and their use. Various knowledge from different fields of humanities and social science will be applied (ethics, communication studies, philosophy of techniques) and will guide the development of technical solutions, as well as recommendations for the implementation of ethical and sustainable AI.
For this reason, we will need both technical and philosophical research, working towards interdisciplinary integration.
The research project is focused on the synthesis of medical images using deep learning, towards better artifact correction and the avoidance of unnecessary medical procedures.
The neural networks designed in this project have a flexible architecture enabling the image synthesis from only an heterogeneous subset of input modalities. The images are synthesized in pathological situations, such as Alzheimer's disease and brain cancers.
This project is part of the effort to prepare Opal for use by patients, providing them with self-management resources such as questionnaires and educational materials.
This project is centered on the data donation aspect of Opal and will involve a Privacy Impact Assessment of the application and the eventual practice of data sharing driven by it.
Multipoint scintillation detectors are used to measure the dose of radiation deposited simultaneously at many locations in space and they have the advantage to allow real-time measurements. However, this detector must be precisely calibrated to provide accurate dose measurements.
The goal of this project is to develop an automated routine for the calibration of multipoint scintillation detectors under the beam of a linear accelerator such as the ones used for cancer treatments, by representing the calibration data in the principal component space.
A multipoint scintillation detector measures the spectrum of the light produced within the detector. Indeed, light is produced within the detector proportional to the radiation deposited in the detector. From a calibration dataset, a Non-Negative Matrix Factorisation algorithm (NMF) is used with the aim to retrieve the pure spectral components of the measurements. To simplify the visualization of the calibration dataset, the dataset is transformed using the Principal Component Analysis algorithm (PCA), and this transformed dataset is then represented graphically in the principal component space. This space allows to visualize the spectral composition of the data, relative to the pure spectra.
Many datasets can therefore be built, represented into this space, and used with the NMF algorithm with the aim to evaluate the performance of this algorithm for different calibration datasets.
In the end, this will allow to determine the experimental datasets that have to be acquired to perform an accurate calibration of the multipoint scintillation detectors.
Discover
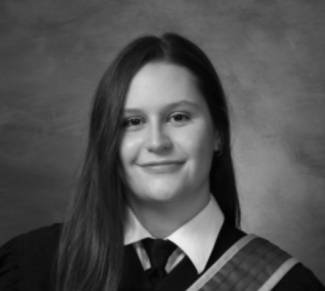
Featured project
Delirium is a condition that, when left unmanaged, is associated with increased mortality and longer hospitalization of patients in intensive care; therefore, its detection should be an integral part of care. It is characterized by confusion, anxiety and reduced alertness. It is estimated that 75% of delirium cases are not detected on admission to hospital. Detecting such an acute condition requires frequent monitoring of participants, which is labor intensive and requires expertise.